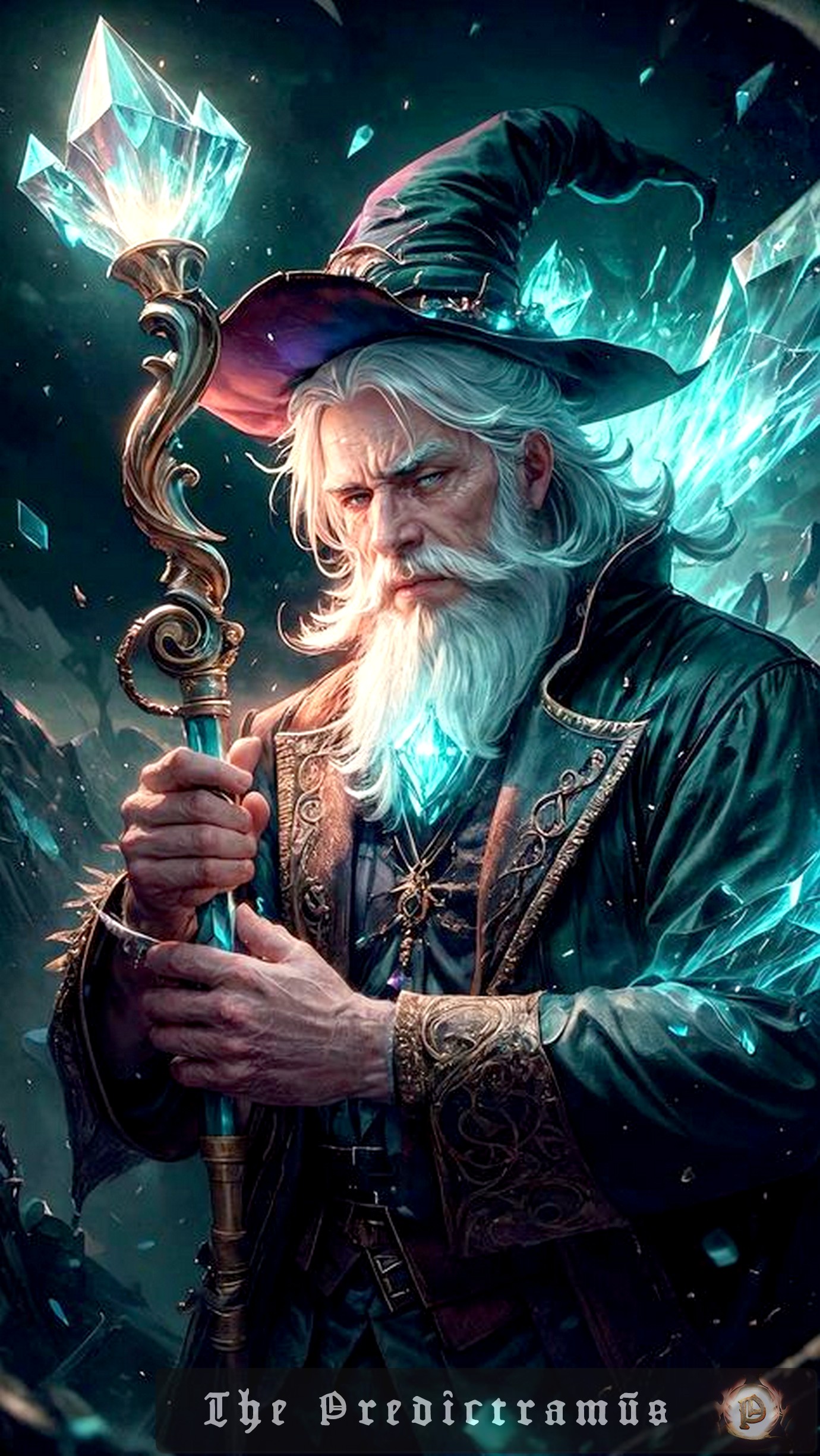
AI Models at Risk of “Model Collapse”: A Threat to the Future of Artificial Intelligence
- No Comments on AI Models at Risk of “Model Collapse”: A Threat to the Future of Artificial Intelligence
- IT
- Forecast for 6 months: In the next 6 months, we can expect to see a growing awareness of the model collapse issue among AI researchers and developers. This may lead to a surge in research and development of new methods to mitigate the problem, such as the use of qualitative and quantitative benchmarks of data sourcing and variety.
- Forecast for 1 year: Within the next year, we may see the first instances of model collapse in real-world applications, such as language models and image generators. This could lead to a re-evaluation of the current AI development paradigm and a shift towards more robust and diverse training data.
- Forecast for 5 years: In the next 5 years, we can expect to see significant advancements in AI research and development, driven by the need to address the model collapse issue. This may lead to the development of new AI architectures and training methods that are more resistant to the problem. However, it may also lead to a widening gap between the capabilities of AI models and their limitations.
- Forecast for 10 years: In the next 10 years, we may see the emergence of a new generation of AI models that are designed to be more robust and resilient in the face of model collapse. These models may use novel approaches to learning and inference, such as hybrid symbolic-connectionist architectures or cognitive architectures that incorporate human-like reasoning and decision-making. However, it is also possible that the model collapse issue may become a persistent problem, limiting the progress of AI research and development.